- Subscribe to RSS Feed
- Mark as New
- Mark as Read
- Bookmark
- Subscribe
- Printer Friendly Page
- Notify Moderator
About Matthew: Matthew is a Data Scientist at C2FO in Kansas City. He previously studied Physics for his BS at the University of Notre Dame followed by the University of Kansas for his MS. When he is not programming, Matthew enjoys playing board games, especially Race for the Galaxy.
Intro
During my time as a Data Scientist, I have been primarily a Python user. However, I wanted access to some of the power offered by R, specifically the auto.arima function in the R forecast package. This post will go over how to get you started on incorporating R functionality into your python workflow.
Want to follow along?
Download Rodeo and head to the tutorials section to find the code!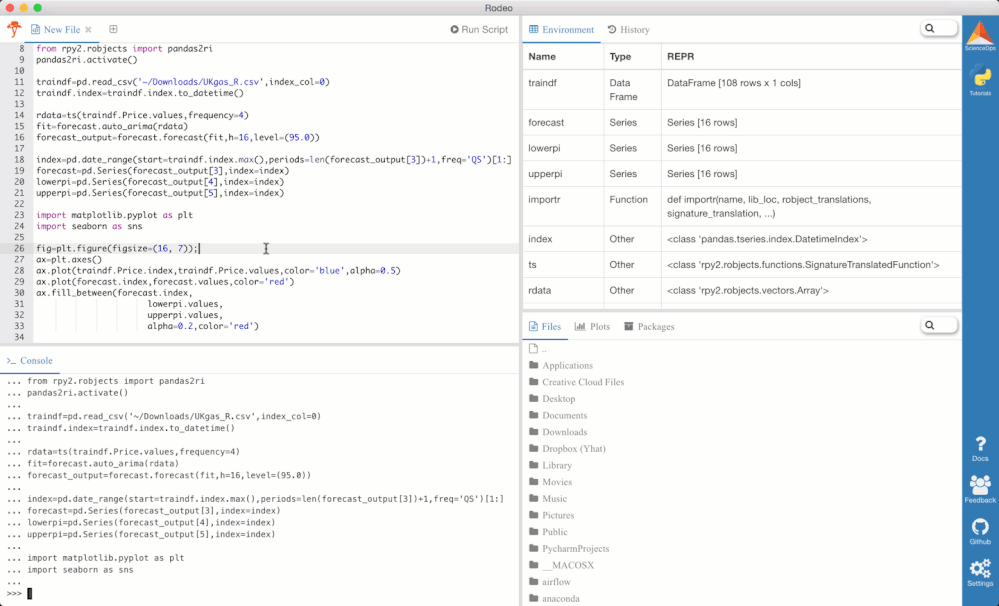
Download Rodeo and follow along!
Python and R: What do they each offer?
The two most popular options for data analysis and modeling are R and Python. Each has their own unique strengths and weaknesses, many which drive a user or a team to choose one over the other. Here are a few key strengths of both that I find particularly valuable:
Python:
- Python is a 'real' programming language, allowing for more flexibility in your ability to solve specific problems
- It offers many other libraries in addition to those needed for a Data Scientist's models
- Python is making strides in the data analysis space with pandas, statsmodels, and scikitlearn
R:
- R libraries have been battle tested far longer than Python, giving a Data Scientist a verified set of tools at their disposal.
- There are also many implementations of various functions, allowing you to find the library that is right for you.
- Due to the long history of R packages, there is a strong community around data analysis.
How Does RPy2 come into play?
RPy2
creates a framework that can translate Python objects into R objects, pass them into R functions, and convert R output back into Python objects. There are many ways that a user can integrate this into their workflow. You may decide to call R library functions as you would native Python functions, or you may decide to write a single R script to run your data on. Below I'll go over the few basics of how to get used to the flow of RPy2, using some sample data from R. We will load in some data, model it in R, and plot the results back in Python.
Note: While I will be working with time series data, I will not be passing them back and forth between Python and R. This can get really tricky and can cause many headaches, so I find it easier to handle all the time series indexing on the Python side.
Importing R objects and libraries to Python
We can import both R functions and libraries as Python objects. We can load a default R function like ts()
through the robjects.r()
function, and assign it to a Python variable. Similarly, we can use importr
to load an R library into a namespace.
import rpy2.robjects as robjects
from rpy2.robjects.packages import importr
ts=robjects.r('ts')
forecast=importr('forecast')
Now that we have these objects in loaded, we can call them similar to standard Python practices. Let's load up some data to model, and create a forecast off of it. You can download the dataset here.
import pandas as pd
from rpy2.robjects import pandas2ri
pandas2ri.activate()
traindf=pd.read_csv('UKgas_R.csv',index_col=0)
traindf.index=traindf.index.to_datetime()
rdata=ts(traindf.Price.values,frequency=4)
fit=forecast.auto_arima(rdata)
forecast_output=forecast.forecast(fit,h=16,level=(95.0))
Note: Take care of the import of pandas2ri
and the activate()
function. These a key to transforming certain datatypes from Python to R.
We've taken our data, transformed it into an robject, and called R functions on our objects. However, we are left with one really messy issue, and that is the output of our function. Our R forecast object isn't nicely translated into a neat Python object for you to parse. You can find all the information from your forecast that you need as shown below:
index=pd.date_range(start=traindf.index.max(),periods=len(forecast_output[3])+1,freq='QS')[1:]
forecast=pd.Series(forecast_output[3],index=index)
lowerpi=pd.Series(forecast_output[4],index=index)
upperpi=pd.Series(forecast_output[5],index=index)
import matplotlib.pyplot as plt
import seaborn as sns
fig=plt.figure(figsize=(16, 7));
ax=plt.axes()
ax.plot(traindf.Price.index,traindf.Price.values,color='blue',alpha=0.5)
ax.plot(forecast.index,forecast.values,color='red')
ax.fill_between(forecast.index,
lowerpi.values,
upperpi.values,
alpha=0.2,color='red')
We can also draw our newly-created plot and save it as a png file on our machine:
Blocking R code into a Function
Instead of bringing everything into Python, we can instead manipulate our objects purely in R, and return only the desired output back to Python. Similar to how we used the robjects.r()
to create a python object mapping to the ts
function, we can define our own function and assign it to a Python object. We still have to create an R data object to pass into the function, but the rest is done on the R side.
rstring="""
function(testdata){
library(forecast)
fitted_model<-auto.arima(testdata)
forecasted_data<-forecast(fitted_model,h=16,level=c(95))
outdf<-data.frame(forecasted_data$mean,forecasted_data$lower,forecasted_data$upper)
colnames(outdf)<-c('forecast','lower_95_pi','upper_95_pi')
outdf
}
"""
rfunc=robjects.r(rstring)
rdata=ts(traindf.Price.values,frequency=4)
r_df=rfunc(rdata)
We now have our resulting forecast in an R Dataframe! You (hopefully) have seen the pandas2ri import above, as this adds a nice easy finish to our data transformation. With its ri2py()
function, we can convert our R Dataframe to a Pandas DataFrame object.
forecast_df=pandas2ri.ri2py(r_df)
forecast_df.index=pd.date_range(start=traindf.index.max(),periods=len(forecast_df)+1,freq='QS')[1:]
forecast_df
forecast | lower_95_pi | upper_95_pi | |
---|---|---|---|
1970-04-01 00:00:00.000001 | 1201.895643 | 1132.969980 | 1270.821305 |
1970-07-01 00:00:00.000001 | 651.095643 | 581.999757 | 720.191529 |
1970-10-01 00:00:00.000001 | 385.395643 | 316.129953 | 454.661333 |
1971-01-01 00:00:00.000001 | 820.795643 | 751.360563 | 890.230723 |
1971-04-01 00:00:00.000001 | 1239.891286 | 1138.581601 | 1341.200971 |
1971-07-01 00:00:00.000001 | 689.091286 | 587.318843 | 790.863728 |
1971-10-01 00:00:00.000001 | 423.391286 | 321.158180 | 525.624391 |
1972-01-01 00:00:00.000001 | 858.791286 | 756.099584 | 961.482988 |
1972-04-01 00:00:00.000001 | 1277.886929 | 1148.555295 | 1407.218562 |
1972-07-01 00:00:00.000001 | 727.086929 | 596.940390 | 857.233467 |
1972-10-01 00:00:00.000001 | 461.386929 | 330.430556 | 592.343302 |
1973-01-01 00:00:00.000001 | 896.786929 | 765.025699 | 1028.548158 |
1973-04-01 00:00:00.000001 | 1315.882572 | 1159.908983 | 1471.856160 |
1973-07-01 00:00:00.000001 | 765.082572 | 607.908555 | 922.256588 |
1973-10-01 00:00:00.000001 | 499.382572 | 341.017226 | 657.747917 |
1974-01-01 00:00:00.000001 | 934.782572 | 775.234792 | 1094.330351 |
Great! Our output is organized nicely into a nice, neat DataFrame, ready to be consumed by all our other Python tools. Now let's plot it to ensure we get the same results...
fig=plt.figure(figsize=(16, 7));
ax=plt.axes()
ax.plot(traindf.Price.index,traindf.Price.values,color='blue',alpha=0.5)
ax.plot(forecast_df.index,forecast_df.forecast.values,color='red')
ax.fill_between(forecast_df.index,
forecast_df['lower_95_pi'],
forecast_df['upper_95_pi'],
alpha=0.2,color='red')
And there we go! That's it!
RPy2 is a fantastic tool that brings the leverage of R functions into a Python workflow. Unfortunately, it is not optimized to return all R objects into a nicely wrapped Python object, though you can write some tools to do so. However, using the method of a self defined R function, you can easily pass in some data you want to analyze, run it through the R functions, and return what you need to Python where its ready to be consumed for future use.
You must be a registered user to add a comment. If you've already registered, sign in. Otherwise, register and sign in.